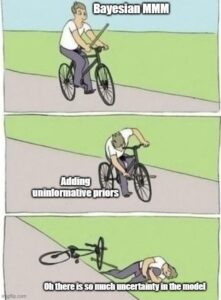
The word uncertainty means different things in Bayesian MMM vs Frequentist MMM.
From a Frequentist perspective, the uncertainty is due sampling variability. That is, there is a true fixed parameter of the population. But given the sample at hand, you may or may not capture this true parameter.
In MMM parlance, one could think of a true ROI of medium as that of a fixed parameter. But because we create our models on samples, chances are that our models may not capture this true ROI accurately. Hence a more representative and adequate sample is always warranted.
From a Bayesian perspective, the uncertainty is not due to the sampling variability, but due to inherent variability in the parameter values themselves.
Bayesian don’t believe in the concept of ‘fixed’ parameters. They think of parameters like it is ‘Whac-a-mole’ game.
Talking about uncertainty in much more detail, there are two types of uncertainties:
📌 Aleatoric uncertainty: It is the inherent uncertainty present in the data. We generally can’t do anything about Aleatoric uncertainty because well it is inherent.
Frequentist MMM modelers refer and care about this uncertainty.
📌 Epistemic uncertainty: Epistemic uncertainty is caused because of lack of knowledge.
While good priors can reduce Epistemic uncertainty, bad or uninformative priors can exacerbate Epistemic uncertainty.
The problem with Bayesian MMM is that (whether they like it or not), the Aleatoric uncertainty is always there. Plus through prior defining, there is always a risk of increasing the epistemic uncertainty too. Thus in Bayesian MMM you have Aleatoric+ Epistemic uncertainty.
📌 Why is Frequentist MMM view of uncertainty better?
Frequentists quantify uncertainty through confidence intervals. Confidence Interval is all about coverage.
That is, if one ran the same statistical test 90 times or 95 times by taking different samples and constructed a confidence interval each time,
would they find the parameter of interest in those intervals each time.
I like to think of MMM as an apparatus that is trying to capture the true Marketing ROI. The data that goes into modeling is a sample from the marketing reality (population).
This data contains the true marketing ROI and your MMM is the experiment that either captures the true marketing ROI or not.
Now as a client, you would want to know how well this apparatus (MMM) is constructed and does it capture the true marketing ROI most of the time?
Confidence Intervals thus in a way informs you about the construct of the MMM model and its reliability.
What about credible interval ?
Well credible interval tells you the probability with which the parameter of interest lies in the particular interval.
Now this does not tell us anything about the build quality of the MMM process or design of experiment in general.