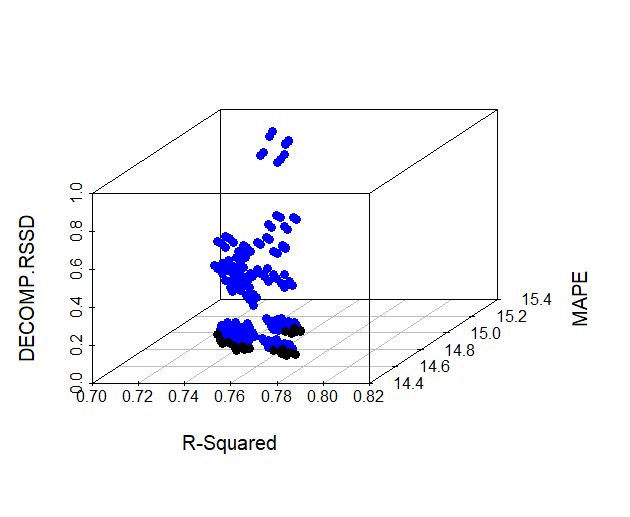
Innovating with Robyn
At Aryma Labs we constantly try to push the frontier of what’s possible in Marketing Measurement and Attribution. In this regard, we have been working on a very interesting problem for a long time. What is this problem? Well, basically your MMM model should not be just good at prediction but should also have good goodness of fit (inference) or vice versa. The question then arises, can we have a model that is good at both? We hence decided to choose models based on 3 metrics – R squared value (provides goodness of fit), Decomp RSSD ( provides business goodness of fit – see link in resources) and finally MAPE