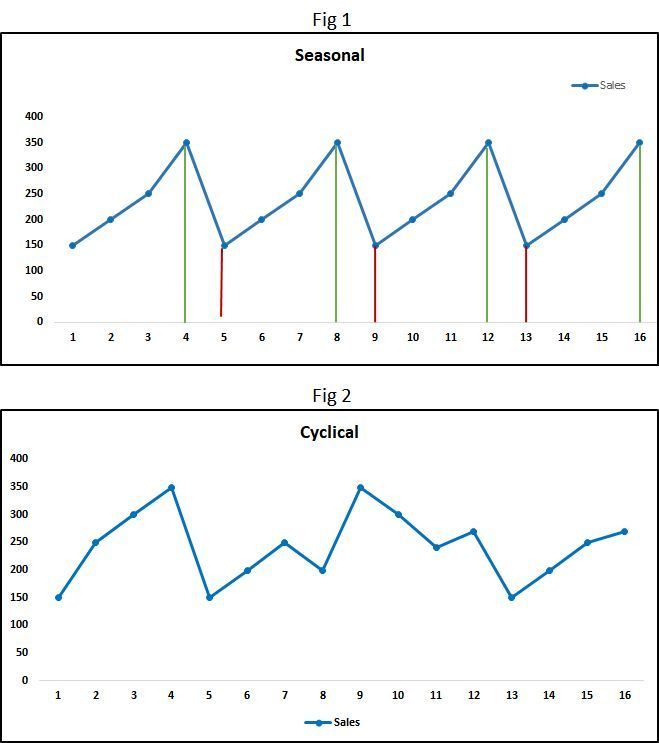
How understanding seasonality and cyclicity can help you build better MMM models
Seasonality ≠ Cyclicity Be it time series analysis or Marketing Mix Models (MMM), the distinction between seasonality and cyclicity is important. Many confuse seasonality with cyclical time series. Here is a quick distinction. Seasonal: A seasonal pattern is a fluctuation which occurs at regular time intervals. These time intervals are predictable. Seasonality does not always refer to changing seasons. It could be anything which happens at predictable and regular intervals. The frequency of these events is fixed and is associated with calendar events. E.g., electricity demand pattern or sales trend showing surges around holiday season. Cyclical: A cyclical pattern is a time series which does not occur at regular intervals.